By Nathan Brannen on 2 October 2024
Proptech is currently experiencing a data revolution. Recent advancements in AI have resulted in unprecedented levels of new information, while insights that were once mere ideas, such as a standardized analysis of every property’s condition or quality, are now widely available.
However, with great power (or great quantities of data), comes great responsibility. As these data sources are being widely used in pricing models and purchase decisions, it is paramount that they are reliable, accurate and explainable.
Companies must be able to trust AI and understand when its predictions should be treated as facts versus a best educated guess. That’s why we created the industry’s first AI confidence scores designed to provide explainability of a property’s photo-based condition and quality. Now, our clients will be able to easily differentiate between a robust score coming from a property with clear photos of all the major areas of a property, and a more uncertain score generated from a single blurry photo.
1. Why Confidence Scores Are Essential for AVMs
As Automated Valuation Models (AVMs) continue to grow in sophistication, it is essential they scale efficiently. They must balance improving the average property valuation across the board, or in AVM terminology, reducing the Mean Absolute Error (MAE), but also ensure there are as few big misses as possible.
Why? The big misses are the ones that have the most painful impact. An agent faces an uphill battle if their client has been anchored to a Zestimate that is 20% higher than what their property is actually worth, while a homeowner is going to be set up for disappointment if they try to get a home equity loan and find out their home is worth a fraction of their AVM influenced expectations.
Unfortunately, up to this point, Restb.ai clients and their AVMs had no way to differentiate between the scores where the AI had a rock solid opinion of a home and those where there was a large degree of uncertainty, which could easily occur due to one of the reasons highlighted in the following section.
Our new confidence score directly addresses this challenge, offering users a transparent, easy to understand value that indicates how reliable a given property condition or quality score is. Feeding the confidence score into a valuation model alongside its corresponding condition and quality allows the AVM to determine how much weight or relevance to place on the property’s scores. This is critical for AVMs, where slight misjudgments in home conditions can lead to significant errors in property valuations.
2. What Factors Influence Confidence?
Working with many of real estate’s leading valuation companies, Restb.ai regularly audits when there have been inconsistencies in an AI generated score and a property’s true condition or quality. In the early days of our models, this would often be related to our models struggling with atypical properties, such as a recently built Victorian home, designed to look decades older, being scored as poor condition due to the styles' more dated origins.
However, more recently, we’ve noticed the majority of inconsistencies are related to the photos included in a listing not clearly or accurately depicting the property for one reason or another.
The key to confidence lies in knowing when the AI is facing one of these “corner case” situations. Based on our experience, here are a few of the factors that can reduce the confidence in a score:
- Number and coverage of photos: What happens when a listing only includes a single exterior photo? While our model’s analysis is accurate based on the information displayed in that photo, a lower confidence score is needed to reflect the uncertainty of not being able to analyze the interior. Alternatively, the more images provided of the entire property, the better our model’s confidence.
Additional photos allow the model to be more confident
- Low quality images: While Restb.ai has gone to great lengths to train our model over images ranging from professional quality to those taken by an overly caffeinated toddler, low quality images (e.g. blurred, poorly lit, etc.) predictably lead to greater variability. Similar to a human, photos that are clear and well-framed are easier for our model’s to score with greater precision.
Confidence is reduced when the images are difficult to analyze
- Virtually staged or rendered photos: Similar to low quality images, we’ve worked diligently to train out biases on digitally modified images, but neither AI (or humans) can magically guarantee a virtually staged rug isn’t covering up a damaged floor. Our model detects digitally modified images and adjusts the confidence score accordingly to account for possible misrepresentation.
Virtually staged images may obscure defects in a property
- Images of properties under construction: In some markets, it is common for new construction homes to feature photos of homes that are in various stages of being built. Without additional context, our AI could score photos of an unfinished new construction home as “poor” condition, but it would be ingested into an AVM associated with a price reflecting a “perfect” condition. These corner cases make it difficult for an AVM to correctly learn the importance of a property’s condition or quality in determining a property’s value.
Both images above may exist within a new construction listing
3. How Confident Scores Help Reduce Valuation Errors
By providing a confidence score alongside each property condition and quality score, Restb.ai is enabling AVMs to better manage the risk of inaccurate valuations. Our models flag when a property’s score has low-confidence, allowing AVMs and valuation companies to:
1. Minimize risk: Low-confidence scores are instantly identified, helping to avoid noise when training valuation models and mitigate large pricing errors that could have significant financial consequences, particularly in lending or investment scenarios.
2. Provide explainability: In addition to a confidence score, context, such as whether a property is under construction or only has one photo, can be returned. Depending on the source of the low confidence, the predicted condition or quality can be treated in the appropriate way.
3. Improve decision making at scale: At the scale AVMs operate, reducing the frequency of errors, even by a small margin, can lead to significant improvements in performance, reliability and trustworthiness. Confidence scores provide a data-driven way to optimize valuations based on available information.
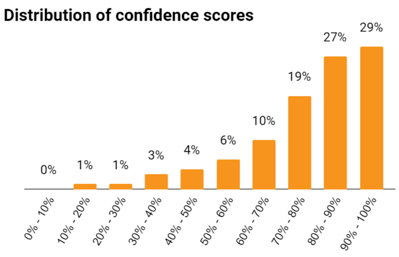
Confidence score distribution across 1 million properties
The Future of Proptech: More Informed, Trustworthy Valuations
At Restb.ai, we believe that providing more transparency and context is essential to the future of proptech and AVMs. As the industry continues to rely on advanced AI models to power everything from home valuations to investment decisions, building trust in those models is indispensable.
Our new confidence score feature is a significant step toward building more reliable, explainable, and scalable real estate technology. By delivering not only the best condition and quality scores but also the confidence to back them up, we’re helping proptech companies around the world make smarter, more accurate decisions—whether they’re analyzing a single home, a local neighborhood or an entire market.
In an industry where even small inaccuracies can lead to costly consequences, the new Restb.ai Confidence Scores represent the next step in refining and improving the accuracy of AVMs, making them more reliable, trustworthy, and ultimately, more valuable.
Have thoughts or questions about our new confidence scores?
Get in touch with us to learn more about how this feature can enhance your AVM and valuation solutions!
comments